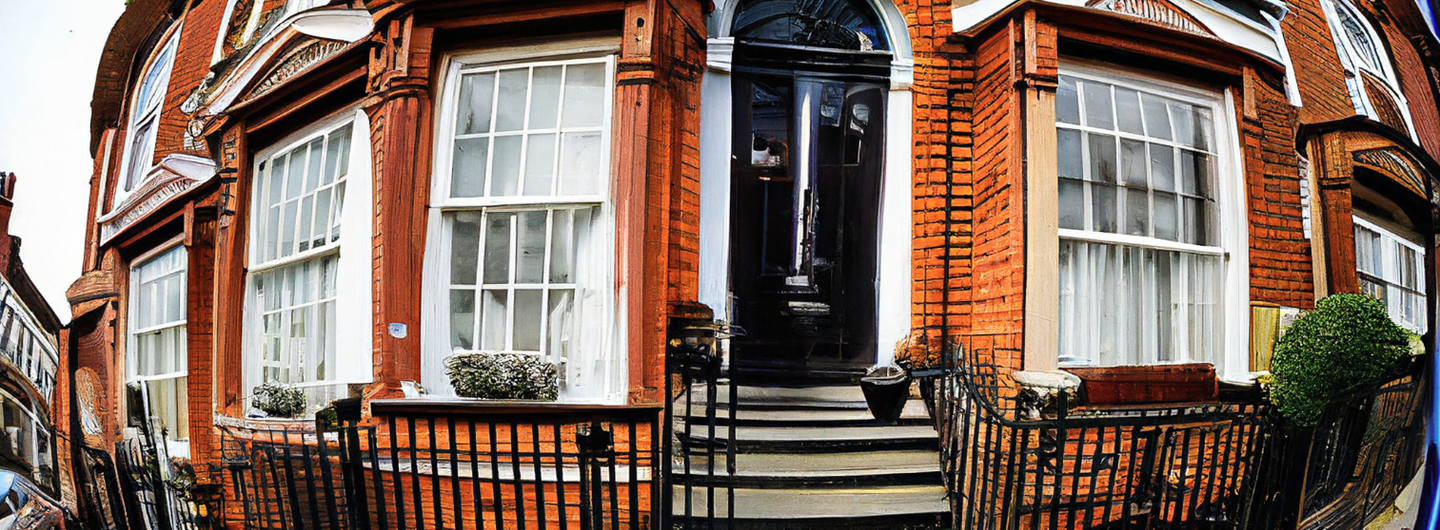
Exploring the potential of machine learning tools to integrate visual data in the analysis and assessment of housing design and policy.
At a glance
- A systematic literature review revealed that despite great progress in the computer vision-based analysis of buildings, practical applications in architecture and urban planning remain rare.
- One of the key barriers to applicability is data integration: it is difficult to create a large-scale visual database of buildings linked to external identifiers.
- We designed a method to create such a database from publicly available street view imagery while being robust to geolocation errors and visually similar buildings.
- The method was evaluated in various areas of London featuring long rows of narrow, visually similar terraced houses – a particularly challenging setting for previous visual indexing techniques.
Key details
More information
The challenge
Advances in deep learning have allowed researchers to tap into the potential of visual data to infer attributes of buildings such as age, style or value. While this is useful to automate time-consuming image-based tasks, multimodal applications that combine visual data with more traditional data sources are still lacking. This is in part due to the difficulty of reliably identifying buildings in images at a large scale.
Street view imagery (SVI) is now a widespread data source for many computer vision-based approaches to urban and architectural analysis. However, SVI presents various issues (geolocation errors, occlusions, etc.) that affect the accuracy of building analysis and the ability to find usable building views. Visual identification is particularly difficult in urban areas featuring long rows of narrow, architecturally similar terraced houses, which are common in the UK.
Our approach
We cast visual building identification as an alignment problem between geolocated SVI and a building footprint map. We formulated a global optimization that refines the location and orientation of building views so that their viewing direction matches the intended building footprint. Multiple views per building were acquired to tackle occlusions due to cars, trees or other buildings.
We fine-tuned two convolutional neural networks (CNN) to increase the robustness of the process. The first CNN is an object detector used to locate individual buildings in each image. The second CNN is a similarity estimator that matches views of the same building while distinguishing between neighbouring buildings.
Outputs
Małgorzata B. Starzyńska-Grześ, Robin Roussel, Sam Jacoby, and Ali Asadipour. 2023. Computer vision-based analysis of buildings and built environments: A systematic review of current approaches. ACM Computing Surveys. https://doi.org/10.1145/3578552
Robin Roussel, Sam Jacoby, and Ali Asadipour. 2023. Finding building footprints in over-detailed topographic maps. Geographical Information Science Research UK (GISRUK). [Poster]
Robin Roussel, Sam Jacoby, and Ali Asadipour. StreetID: Robust multi-view indexing of buildings from street-level images. [Under review]
Team
Ask a question
Get in touch to find out more about our research projects.
[email protected]
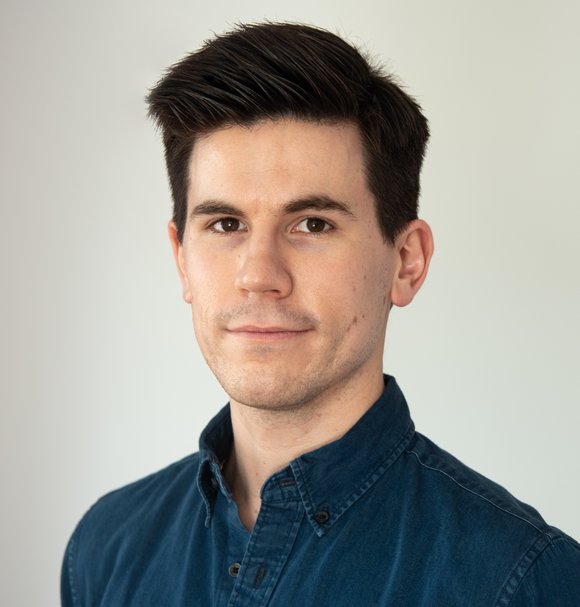